Moving from data collection to understanding and application.
What I study and how I am studying it are strikingly similar. Teachers and students, as well as myself, engage in data collection and analysis to make meaning and guide future work. This post highlights some similarities and outlines my data analysis tools and strategy.
I focused my dissertatin on the collection and use of data in personalized classrooms by students and teachers. The tools and processes I discovered in the classroom are strikingly similar to those I’ve taken to collect and analyze data for this dissertation. In the classroom, students and teachers collect data related to a shared goal they have identified; in my dissertation, I collect data related to my research questions. Students and teachers use tools to engage in analysis of the data and create meaning; in my dissertation, I do the same. Where we differ – at least for now – is in how we use our findings. Students and teachers in classrooms work together to use their findings from the data to create an action plan towards further interest- and standards-based learning. My action plan is to influence the future or formal learning environments itself.
Collecting and analyzing data to understand data use in personalized learning.
Ha! That’s a lot of data. Sorry about that. Said in another way, I am collecting empirical evidence of how teachers and students engage in data use in personalized learning classrooms. Aristotle identified three kinds of knowledge – scientific, technical, and practical wisdom. This dissertation highlights the last; it focuses on documenting the practical wisdom of educators in the field who are designing and implementing data-use strategies with students.
A summary of the steps I took to get here:
- Establish research question – How do students and teachers collect and use data in personalized learning settings?
- Literature Review – Read and comprehend literature in two primary domains: personalized learning and school data use.
- Theoretical Framework – Based on findings from literature review, establish a theoretical framework that will focus and guide inquiry. I chose to modify a framework established by Halverson et al. – Data Driven Instructional Systems – within the context of personalized learning.
- Research Design – Because I wanted to know how teachers and students used data in PL classrooms, I chose to find and examine three exemplary cases likely to provide useful information for my study. I leaned on my experience through a pilot study and the recommendation of regional experts to identify cases to study.
- Data Collection – Based on my research question and theoretical framework, I created interview and observation protocols to collect pertinent information.
- Data Analysis – I created a list of codes based off of the literature, pilot study, and early findings from my sites. I reviewed and made notes on all my data and then went through and coded it twice. I used a book by Miles & Huberman to guide me through this process.
The process was not entirely linear. I went back and forth between the literature and subsequent steps often during the research process.
MaxQDA helped me to organize, memo, and code my data. Consider MaxQDA and similar tools like NVivo as cognitive aids. You could do many of the same things with printed data, pocket folders, highlighters, and post-its (as my professor and friend Clif Conrad might remind you). Below is a set of codes I used to analyze my data in my dissertation research and what they mean.
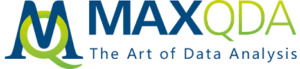
Analysis to understand data use in personalized learning environments
Personalized Learning Codes (derived from literature and the pilot study)
- Personal learning plan
Use this code when you notice tools or routines involved in creating or modifying the goals or means of individual student learning. - Personal learning profile
Use this code when you notice tools or routines involved in documenting student data (both student- and standards-centered). - Flexible learning environment
Use this code when you notice routines or tools or aspects of the environment that creates flexibility in the time, pace, manner, or place of learning. - Culture of student agency
Use this code when you notice students making decisions or when you notice routines or tools that enhance student agency. - Conferring
Use this code when you notice tools or routines that facilitate interaction between individual students and teachers and individual student data.
Data Driven Instructional Systems (derived from literature and work by Halverson et al.)
- Collection Use when a source of data or routine of data collection is noticed.
- Reflection Use when a routine or tool that aids in making sense of data, analyzing data, or finding insight through data is noticed.
- Program design Use when a routine or tool that aids in program design is noticed.
- Formative feedback Use when formative feedback tools or routines are noticed.
Data Sources (derived from first round of coding)
- Student-centered
Use this code when you notice the collection or use of student-centered data. - Standards-centered
Use this code when you notice the collection or use of standards-centered data.
You can see early thoughts and work from this dissertation research in other posts on this site. A takeaway from this work is that we don’t have to make students wait until they are in graduate school to guide the collection and analysis of their own data towards interest- and inquiry-driven learning goals. We can and should start building in our students the skills and habits required to engage in inquiry much, much sooner.